Time Series Analysis
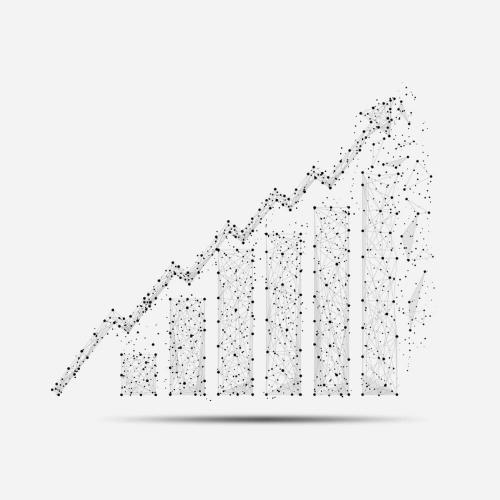
We created this program for those of you, who are interested in working with time series data.
During the first semester, we focus on the essential fundamentals, such as basic concepts of math and programming, which are the same for all programs.
In the Math & Statistic course, students will learn about discrete mathematics and the basics of probability theory. Algorithms & Data Structures covers the basic algorithms on graphs, data structures, and recurrence relations. Also, during the first semester, students learn how to process and analyze data, and build simple models based on this data in the Data Science course. In the Advanced Programming Languages course, students will learn about programming languages and their fundamental differences.
During the second semester, students will get acquainted with the basics of machine learning, learn how the main machine learning models work (in the Machine Learning course), and also try to use them in practice on complex tasks in the Data Science course (part 2). Students of this program will also take a Systems Architecture training course.
The major course of this program is Practical Time Series Analysis. We will offer students to put into practice their knowledge of algorithms for constructing and analyzing time-series data, including, but not limited to, analysis of various indicators of the financial markets.
Semester 1
Math & Statistics
Set Theory
Relations and Functions
Logic and Induction
Sequences
Number Theory
Combinatorics
Recurrence relations
Counting techniques
Graph Theory
Graphs and Numbers
Growth of Functions
The Probability of an Event
Discrete Random Variables
Continuous Probability
Conditional Probability
Distributions and Approximation
Sampling Theory
Statistics
Advanced Algebra
Algorithms and Data Structures
Sorting and Searching
Graphs (basics)
BFS and DFS
Dynamic Programming
LIS, LCS and other 2D problems
Heaps
Dijkstra, Floyd, Bellman-Ford
DFS applications
Knapsack problem
Dynamic programming over subsets
Amortized Time Complexity (Queue via Stack)
Disjoint Set Union
Dynamic programming on a tree
Data Structures. RSQ/RMQ, Sqrt Decomposition, Sparse Table
Minimum Spanning Trees
Matching
Segment Trees
Trie
Binary Search Trees
LCA
What is NP and how is it useful?
Strings
Data Science
AI Introduction
Machine Learning Basics
ML as a task of optimization
Hyperparameters
Probabilistic Approach in ML
Models Validation
Linear regression
Logistic regression
Decision tree
SVM algorithm
Naive Bayes algorithm
KNN algorithm
K-means
Random forest algorithm
Dimensionality reduction algorithms
Gradient boosting algorithm and AdaBoosting algorithm
Data Preprocessing
Time Series Forecasting
Ranking task
Advanced Programming Languages
The dawn of programming
Grammars
Programming Language Spectrum
Programming paradigms
Programming language syntax
ANTLR
Compilation and interpretation
Binding and memory management
Mutable vs. immutable data structures
Data-Oriented Programming
LLVM
Linking
Introduction to Clojure
Clojure macros
Languages for data
Constraint programming
Lua
Elixir
Learning and coding with LLMs
Semester 2
Databases overview
Introduction to Databases and the Industry Landscape
Practical Data Modeling Across Paradigms
Setting Up the Database Environment
Relational Databases. MySQL and PostgreSQL Basics
Analytical Databases with DuckDB and Advanced SQL Techniques
Python ORMs. Using SQLAlchemy and Django ORM
Schema Migrations with Alembic
NoSQL and Specialized Data Stores
Document Stores. Working with MongoDB
Key-Value Stores. Leveraging Redis
Graph Databases. Exploring Neo4j and Cypher
Wide-Column Stores. Apache Cassandra/HBase
Time-Series Databases. InfluxDB/TimescaleDB
Search Engines as Databases. Introduction to Elasticsearch
Multi-Model Databases. Exploring ArangoDB
Integrating Multiple Databases. Polyglot Persistence Strategies
Real-World Case Studies, Emerging Trends, and Course Wrap-Up
Systems Architecture and Distributed Protocols
C++ - syntax, OOP basics, UB, numbers
Rust - syntax, programming paradigms, safe/unsafe, comparison with C++
Memory Management. Stack and heap memory, variable sizes, Ownership, smart pointers
Memory Management. Core containers, iterators, internal implementations. Error handling
Syscalls, Processes, Scheduling
System limits - rlimit, cgoups, Linux namespaces, seccomp
Multithreading. Mutex, atomic operations. Condition variables, channels
Multithreading. Async functions, coroutines, green threads
Observability. Metrics, Prometheus, Grafana. Logging, telemetry, alerts
Distributed Systems
Data encoding: UTF-8, big-endian vs. little-endian, prefix encoding, possibly Huffman trees
Deserialization: JSON, Protobuf, etc.
Traffic balancing, Nginx
Databases, message queues
Assembler (Instructions, RISC, CISC, x86, Intel, asm basics)
Data Science (part 2)
Big Data, Hadoop, Spark
Artificial Neuron Model
Multilayer ANN 1: Hyperparameters, Regularization, Training Process
Multilayer ANN 2: Adam, Dropout, Weight Initialization, Batch Normalization
Convolutional Neural Networks (CNN)
Recurrent Neural Networks (RNN) – LSTM, GRU
Neural Network Architectures – GAN, Seq2Seq, Autoencoder
Attention and Transformers
Generative AI: Principles and Types of Models
Generative AI: Prompt Engineering, Fine-Tuning, LLM Inference
Approaches to Building RAG Based on LLM
AI Ethics
Practical Time Series Analysis
Introduction to Forecasting
Baseline models
Random Walks
Moving Average Process
Autoregressive Process
Forecasting an Autoregressive Process
Mixed Autoregressive Moving Average Process
Non-stationary Time Series
Seasonality
External Variables
Forecasting Multiple Time Series
Introduction to Forecasting with Deep Learning
Baselines for Deep Learning
Linear Models
Implementing a Deep Learning Network
Long Short-Term Memory Models